
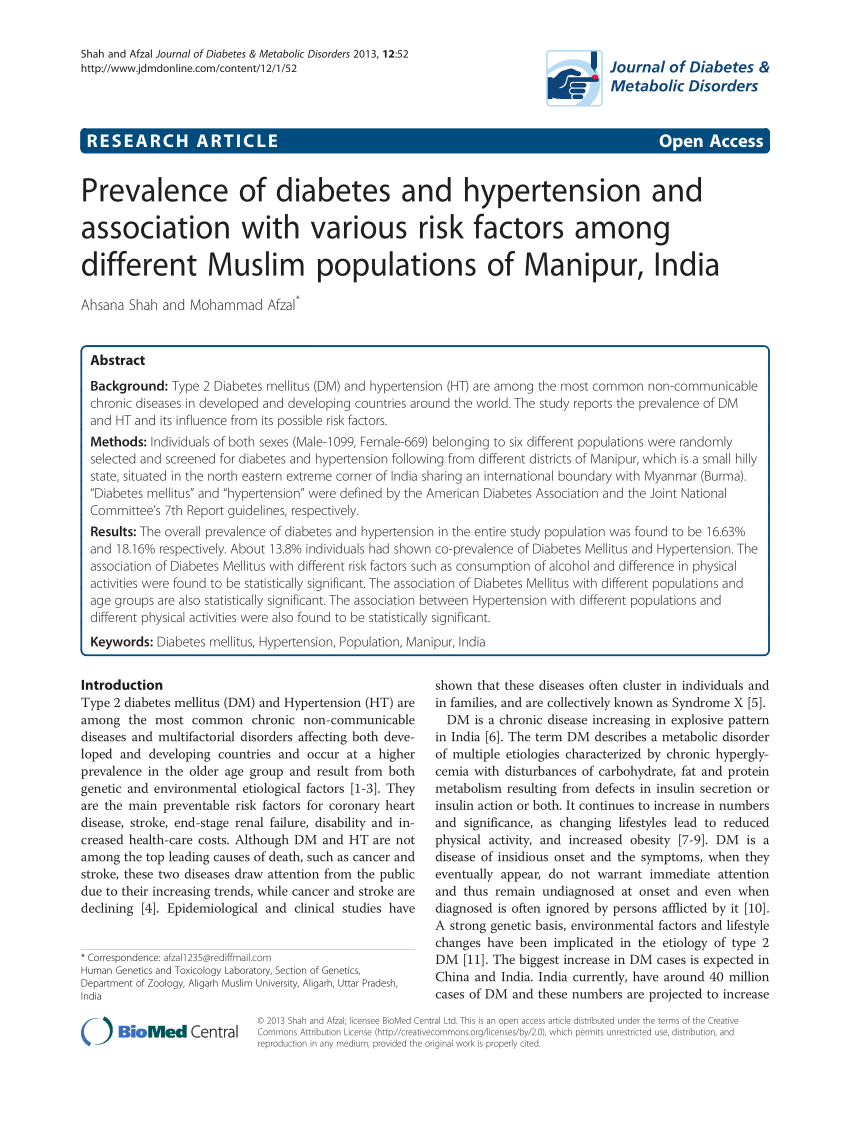
In our analysis using a particular setting of input variables, XGBoost showed that the OGTT variables were more important than fasting plasma glucose or glycated hemoglobin.Ĭonclusions: A machine learning approach, XGBoost, showed better prediction accuracy compared with LR, suggesting that advanced machine learning methods are useful for detecting the early signs of diabetes or GMD.

#Risk prevalence for type 2 diabetes pilot study pdf full
The AUC values increased when the full OGTT variables were included. The machine learning approach using XGBoost showed better performance compared with traditional LR methods. For study 2, the AUC values ranged from 0.63 to 0.78. Results: For study 1, the AUC values ranged from 0.78 to 0.93. The other 6 classification models, D to I, used LR for comparison. Three classification models, A to C, used XGBoost with various input variables, some including OGTT data. For each study, a randomly chosen subset representing 80% of the data was used for training 9 classification models and the remaining 20% was used for evaluating the models. After data processing, 13,5 OGTTs were analyzed for study 1 and study 2, respectively. If a subject was diagnosed with diabetes or GMD at least once during the period, then that subject’s data obtained in previous trials were classified into the risk group (y=1).

For each study, to apply supervised machine learning methods, the required label data was prepared. Given the data, 2 studies were conducted: predicting the risk of developing diabetes (study 1) or GMD (study 2). For each examination, a subject was diagnosed with diabetes or prediabetes according to the American Diabetes Association guidelines. Methods: Data were collected from subjects who underwent multiple OGTTs during comprehensive check-up medical examinations conducted at a single facility in Tokyo, Japan, from May 2006 to April 2017. We compared the performance of the machine learning techniques with logistic regressions (LR), which are traditionally used in medical research studies. The receiver operating characteristic (ROC) curves and their area under the curve (AUC) values for the trained classification models are reported, along with the sensitivity and specificity determined by the cutoff values of the Youden index. Objective: We trained several classification models for predicting the risk of developing diabetes or GMD using data from thousands of OGTTs and a machine learning technique (XGBoost). Complete OGTT information, such as 1-hour and 2-hour postloading plasma glucose and immunoreactive insulin levels, may be useful for predicting the future risk of diabetes or glucose metabolism disorders (GMD), which includes both diabetes and prediabetes. JMIR Bioinformatics and Biotechnology 16 articlesĮmail: A 75-g oral glucose tolerance test (OGTT) provides important information about glucose metabolism, although the test is expensive and invasive.JMIR Biomedical Engineering 59 articles.JMIR Perioperative Medicine 65 articles.Journal of Participatory Medicine 69 articles.
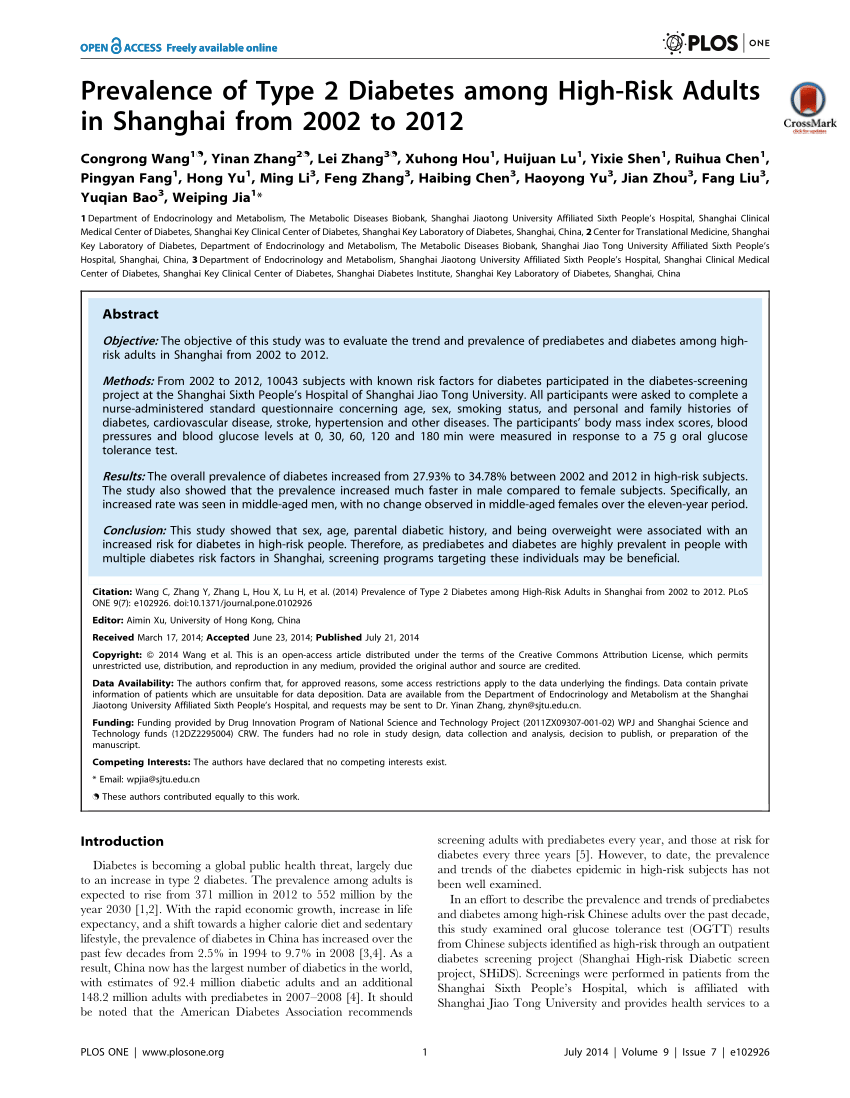
JMIR Rehabilitation and Assistive Technologies 168 articles.JMIR Pediatrics and Parenting 224 articles.Interactive Journal of Medical Research 244 articles.JMIR Public Health and Surveillance 940 articles.Journal of Medical Internet Research 6775 articles.
